
Credit Card Fraud Detection
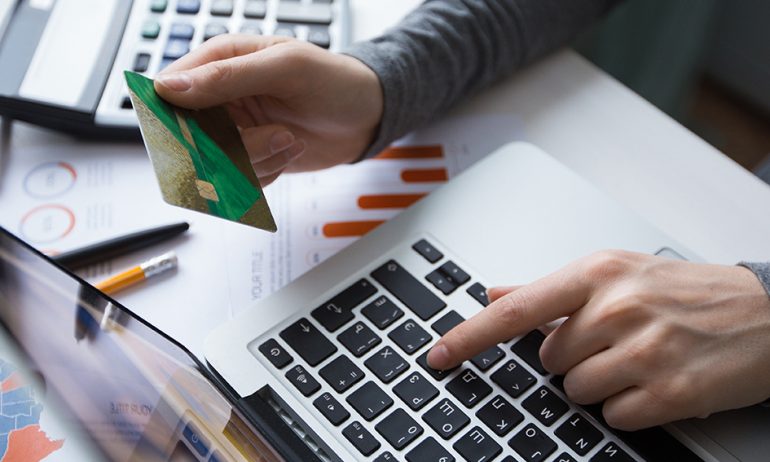
Project URL: https://github.com/Jack-Merrett/credit_card_fraud_detection
Credit Card Fraud Detection
Welcome to the Credit Card Fraud Detection project! This project focuses on building a machine learning model to detect fraudulent credit card transactions. The dataset used for this project can be found on Kaggle.
Introduction
Credit card fraud is a significant concern in the financial industry. This project aims to develop a machine learning solution to identify potentially fraudulent transactions from a dataset containing credit card transactions. By leveraging various machine learning algorithms and techniques, the goal is to create a reliable model that can accurately distinguish between genuine and fraudulent transactions.
Dataset
The dataset used for this project is sourced from Kaggle's Credit Card Fraud Detection Dataset. It consists of a collection of credit card transactions, including both genuine and fraudulent ones. The dataset provides various features such as transaction amount, time, and anonymized numerical features derived from the transaction.
Project Instructions
The main objective of the Credit Card Fraud Detection project is to build and evaluate a machine learning model capable of detecting fraudulent transactions. Here's an outline of the project tasks:
-
Load and Explore the Dataset: Import the dataset using appropriate libraries and explore its contents.
-
Data Preprocessing: Clean and preprocess the dataset. Handle any missing values, perform feature scaling, and split the data into training and testing sets.
-
Model Building: Train and evaluate various machine learning algorithms such as Logistic Regression, Random Forest, Support Vector Machines, etc. Tune hyperparameters to achieve the best model performance.
-
Model Evaluation: Evaluate the models using appropriate evaluation metrics like accuracy, precision, recall, F1-score, and confusion matrix. Pay special attention to the model's ability to detect fraudulent cases.
-
Conclusion and Deployment: Summarize the results and select the best-performing model. Discuss the model's strengths and limitations. If applicable, deploy the model for real-time fraud detection.
Prerequisites
To successfully complete this project, you should have a solid understanding of machine learning concepts and experience with Python programming. Familiarity with libraries like Pandas, NumPy, Scikit-Learn, and Matplotlib will be essential for data preprocessing, model building, and result visualization.
Getting Started
To get started with the Credit Card Fraud Detection project, follow these steps:
- Clone this repository to your local machine.
- Download the Credit Card Fraud Detection dataset from Kaggle and place it in the project directory.
- Open the provided Jupyter Notebook or Python script to begin working on the project tasks.
License
This project's code is released under the Open License. The dataset used in this project has its own licensing terms, which you can find on Kaggle.
Acknowledgments
This project was inspired by the need to tackle credit card fraud using machine learning techniques. Special thanks to Kaggle for providing the dataset and to the open-source community for developing the libraries and tools used in this project.